Data-Driven Recruitment: The Future of Smart Hiring
Hiring top talent is more competitive than ever. Traditional recruitment methods, like relying on resumes and gut instincts, often lead to bad hires, increased turnover, and wasted resources. But what if there was a smarter way to hire?
Data-driven recruitment is transforming hiring by leveraging analytics, AI, and predictive insights to make better decisions. Companies like Google, Amazon, and Unilever are already using data-driven hiring to optimize their recruitment process, reduce bias, and improve efficiency.
In this article, we’ll explore:
✅ What data-driven recruitment is and how it works
✅ The benefits of data-driven hiring
✅ Essential recruitment metrics & tools
✅ Real-world examples & how to implement data-driven recruitment
If you want to stay ahead in the hiring game, it’s time to embrace data-driven recruitment strategies!
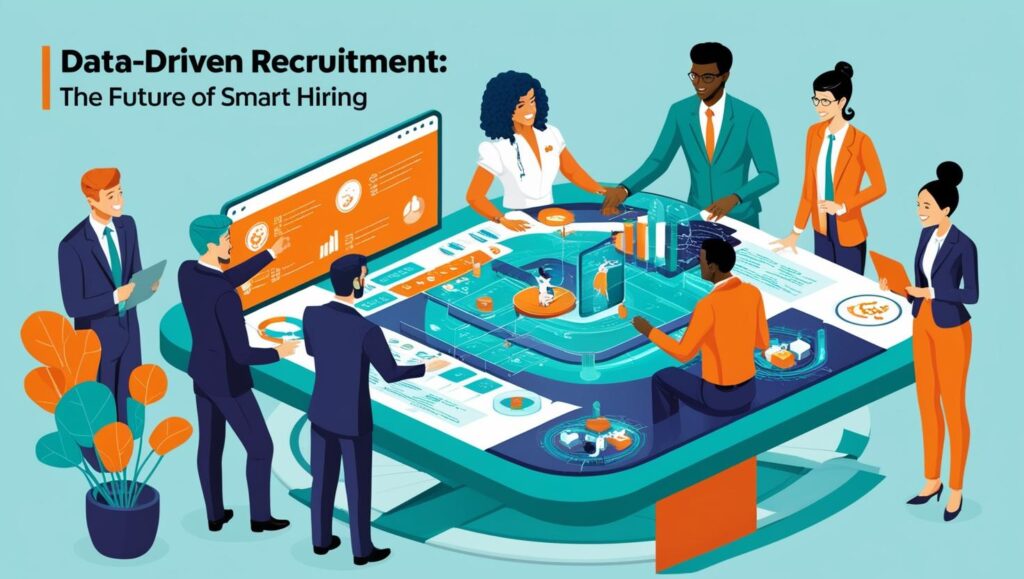
What is Data-Driven Recruitment?
Data-driven recruitment is a modern hiring approach that uses data, AI, and analytics to enhance the recruitment process. Instead of relying on intuition, companies use hard data to make hiring decisions—improving efficiency and reducing hiring mistakes.
Traditional Hiring vs. Data-Driven Hiring
Traditional Hiring | Data-Driven Hiring |
---|---|
Based on resumes & intuition | Uses AI & predictive analytics |
Slow & manual process | Automated & fast hiring |
Prone to bias & errors | Reduces bias with structured data |
No tracking of hiring success | Measures performance with recruitment metrics |
Why is Data-Driven Recruitment Important?
🔹 Improves hiring accuracy by selecting candidates based on proven skills & performance.
🔹 Reduces hiring costs by optimizing job advertisements & eliminating ineffective hiring channels.
🔹 Speeds up hiring with AI-driven resume screening & automated processes.
🔹 Enhances candidate experience by matching job seekers to roles that fit their skills.
📌 Example:
Companies like Amazon and Google use predictive hiring models to analyze candidate data and forecast job performance, reducing hiring errors.
Key takeaway: If you’re not using data-driven recruitment strategies, you’re already falling behind!
Benefits of Data-Driven Recruitment
A data-driven recruitment process offers multiple advantages for both employers and job seekers.
1. Improves Hiring Accuracy: AI and predictive analytics can identify top candidates based on past hiring success.
Hiring managers can use historical data to predict which candidates will perform best.
2. Reduces Hiring Costs: Data-driven hiring helps companies spend less on ineffective job ads.
Tracking cost-per-hire ensures that recruitment budgets are spent efficiently.
3. Speeds Up the Recruitment Process: AI-driven resume screening shortlists top candidates in minutes.
Automated scheduling tools eliminate back-and-forth interview scheduling.
4. Enhances Diversity & Reduces Bias: Data eliminates unconscious bias by focusing on skills instead of subjective factors.
Example: AI recruitment software can remove gender or ethnic indicators from resumes to ensure fair hiring.
5. Improves Candidate Experience: Predictive hiring matches candidates to roles that fit their skills, leading to higher job satisfaction.
Data helps personalize job recommendations and interview processes.
Example: Unilever reduced hiring time by 75% by implementing AI-based video interviews and psychometric testing.
Next, let’s explore the key recruitment data metrics that drive successful hiring!
Key Data Metrics in the Data-Driven Recruitment Process
To build a successful data-driven recruitment strategy, companies must track the right hiring metrics.
1. Time to Fill
Measures how quickly a job position is filled.
Helps recruiters optimize job postings & hiring timelines
2. Cost per Hire
Tracks total spending on recruitment (job ads, ATS software, recruiter salaries).
Helps companies budget better & reduce unnecessary hiring costs.
3. Quality of Hire
Evaluates new hires’ job performance & retention rate.
Uses performance reviews & KPI tracking to measure success.
4. Source of Hire
Identifies the best-performing recruitment channels (LinkedIn, job portals, referrals).
Helps companies focus on high-quality talent sources.
5. Turnover Rate
Measures how often employees leave a company after being hired.
High turnover indicates poor hiring decisions or workplace issues.
Example: Netflix tracks quality-of-hire metrics to ensure that each new hire contributes to long-term business success.
Next, let’s explore the best tools that make data-driven recruitment possible!
Data-Driven Recruitment Tools & Technologies
To successfully implement data-driven recruitment, businesses need AI-powered recruitment tools.
Applicant Tracking Systems (ATS)
Greenhouse – Tracks hiring data & automates recruitment tasks.
Workday – AI-driven recruitment analytics platform.
Lever – Advanced recruitment data tracking & automation.
AI Resume Screening Tools
HI revue – Uses AI to analyze video interviews.
Plyometrics – AI-powered psychometric & behavioral assessments.
LinkedIn Recruiter – AI-driven candidate matching & sourcing.
Predictive Analytics Software
Eightfold AI – Uses AI to predict candidate success.
LinkedIn Talent Insights – Helps recruiters find top talent trends
Example: Unilever uses AI-based assessments and predictive analytics to improve hiring decisions.
Next, let’s discuss the challenges of data-driven recruitment and how to overcome them!
Challenges of Implementing Data-Driven Recruitment & Solutions
While data-driven recruitment strategies are powerful, companies face challenges like:
Data Privacy & Compliance Issues
Ensure hiring tools follow GDPR & data security laws.
AI Bias in Hiring
Use AI models trained on diverse data sets to avoid discrimination.
High Costs of AI Recruitment Tools
Start with free ATS software and upgrade as needed.
Example: Companies like Microsoft & IBM continuously audit their AI recruitment tools to remove bias & compliance risks.
Next, let’s explore the future of AI-driven hiring!
Future of Data-Driven Recruitment
While data-driven recruitment strategies are powerful, companies face challenges like:
The future of hiring will rely on:
- AI-driven hiring assistants for real-time candidate assessments.
- Machine learning models that predict employee success before hiring.
- Blockchain in recruitment to verify candidate credentials securely.
Example: IBM is developing AI hiring algorithms that predict which candidates will stay long-term.
Final Thoughts: Data-driven recruitment isn’t just a trend—it’s the future of hiring!
Real-World Examples of Data-Driven Hiring
Case Study 1: Google – Data-Driven Candidate Evaluation
Problem: Google struggled with long hiring cycles and inconsistent interview decisions.
Solution: They implemented structured interviews, data-backed scoring, and AI-powered screening tools.
Results:
- Reduced hiring time by 40%
- Improved candidate quality by using predictive analytics
Case Study 2: Amazon – AI-Driven Resume Screening
Problem: Amazon receives millions of job applications, making manual screening inefficient.
Solution: They developed an AI-powered ATS (Applicant Tracking System) to scan resumes based on key hiring metrics like skills and experience.
Results:
- 75% faster candidate screening
- Increased diversity by removing human bias in resume selection.
Case Study 3: Unilever – AI-Powered Video Interviews
Problem: Unilever’s traditional hiring was costly and slow, with limited insight into candidate personality and skills.
Solution: They used AI-driven video interviews, where machine learning analyzed facial expressions, word choice, and tone to assess cultural fit.
Results:
- Reduced hiring time by 75%
- Increased new hire retention by 20%
Key Takeaway:
Leading companies leverage AI, analytics, and recruitment data to make faster, smarter hiring decisions.
Step-by-Step Guide: How to Implement Data-Driven Recruitment
Step 1: Define Your Hiring Goals
Identify the key roles that need improvement through data-driven hiring.
Set measurable KPIs (e.g., reducing hiring time, improving retention).
Step 2: Choose the Right Recruitment Metrics
Track important hiring KPIs like time-to-fill, cost-per-hire, and quality-of-hire.
Use AI tools to measure past hiring success and predict future performance.
Step 3: Use AI & Automation Tools
Implement an Applicant Tracking System (ATS) to automate resume screening.
Use predictive analytics software to assess candidate success probability
Step 4: Reduce Bias with Data-Backed Decisions
Train AI models to focus on skills and experience instead of demographics.
Conduct structured interviews where candidates are scored based on predefined data points.
Step 5: Continuously Optimize with Data
Analyze hiring trends and refine recruitment strategies based on data insights.
Use employee performance data to improve future hiring decisions.
Key Takeaway:
Following these five steps, businesses can improve hiring efficiency, reduce bias, and attract top taken.
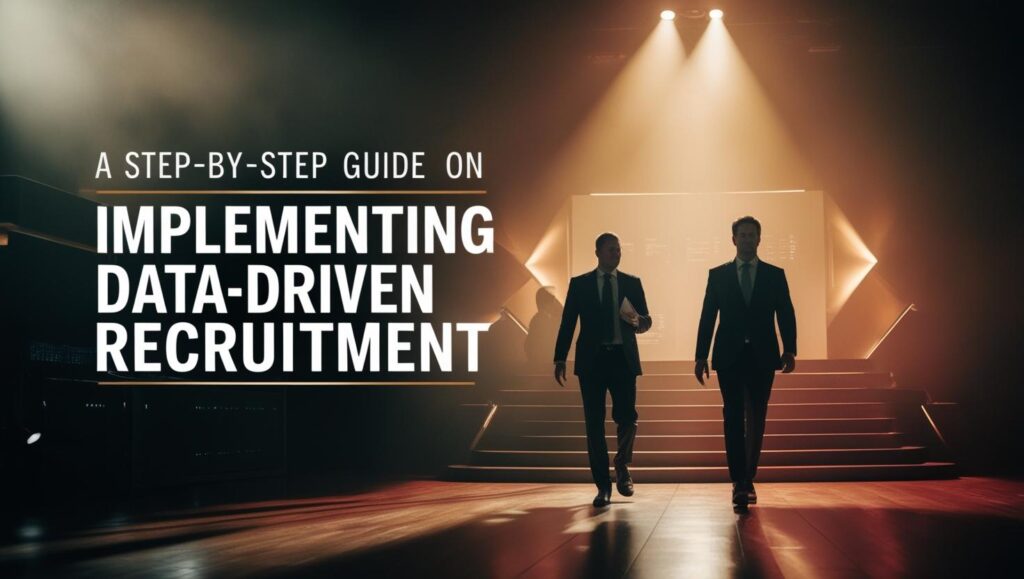
How Data-Driven Recruitment Improves Diversity & Inclusion
The Problem: Bias in Traditional Hiring.
Traditional recruitment often involves unconscious biases, where hiring managers favor certain genders, ethnicities, or backgrounds.
The Solution: Data-Driven Hiring for Fair Recruitment
✅ AI screening tools remove personal identifiers like name and gender, ensuring fair candidate evaluation.
✅ Predictive analytics match candidates based on skills and job fit, not personal characteristics
Real-World Example: IBM’s AI for Bias-Free Hiring
- IBM’s AI-powered hiring tools analyze skills, experience, and cultural fit instead of race or gender.
- Result: More diverse hires and equal opportunities for all applicants.
Key Takeaway:
Data-driven recruitment creates equal hiring opportunities, helping businesses build diverse, inclusive workplaces.
Common Myths About Data-Driven Recruitment (Debunked!)
❌ Myth 1: AI Will Replace Human Recruiters
✅ Reality: AI helps automate tasks but human recruiters are still essential for final hiring decisions and relationship-building.
❌ Myth 2: Data-Driven Hiring Is Only for Large Companies
✅ Reality: Even small businesses can use free ATS tools and basic recruitment analytics to improve hiring.
❌ Myth 3: AI in Hiring Is 100% Unbiase
✅ Reality: AI reduces bias, but it must be trained on diverse datasets to prevent discrimination.
❌ Myth 4: Data-Driven Recruitment Is Too Expensive
✅ Reality: Many low-cost and free tools are available, and data-driven hiring reduces long-term hiring costs.
❌ Myth 5: Data-Driven Hiring Ignores Soft Skills
✅ Reality: AI-driven hiring tools now analyze soft skills using behavioral assessments and AI interviews.
Key Takeaway:
Understanding the realities of data-driven hiring helps businesses make informed recruitment decisions.
Actionable Takeaways & Best Practices
Best Practices for Effective Data-Driven Recruitment
- Use AI-powered ATS tools to speed up resume screening and job matching.
- Leverage predictive analytics to hire candidates with long-term success potential.
- Monitor key hiring metrics (cost-per-hire, time-to-fill, quality-of-hire) for continuous optimization.
- Train HR teams on data-driven hiring tools for better decision-making
- Ensure compliance with hiring laws to avoid legal risks in AI hiring
Final Thoughts: Why Data-Driven Recruitment Is the Future
- Companies using data-driven hiring strategies gain a competitive advantage.
- AI-driven tools help reduce hiring bias, improve candidate experience, and increase hiring efficiency.
- Businesses that ignore data-driven recruitment risk falling behind in talent acquisition.
FAQs
What is data-driven recruitment?
Data-driven recruitment is the process of using AI, analytics, and hiring data to make better hiring decisions instead of relying on intuition.
How does data-driven hiring improve recruitment?
It helps recruiters analyze candidate performance, reduce bias, speed up hiring, and optimize hiring costs.
What are the key metrics in data-driven recruitment?
Important metrics include time to fill, cost per hire, quality of hire, source of hire, and turnover rate.
What are the best tools for data-driven recruitment?
Popular tools include Greenhouse, Workday, LinkedIn Talent Insights, HireVue, and Eightfold AI.
Does data-driven recruitment eliminate hiring bias?
It reduces bias by focusing on skills and performance data, but AI models must be carefully trained to avoid algorithmic discrimination.
Is data-driven hiring only for large companies?
No! Small businesses can also use free ATS software and recruitment analytics to improve their hiring process
What are the biggest challenges of data-driven recruitment?
Challenges include data privacy issues, AI bias, and the cost of implementing recruitment technology.
Can AI replace human recruiters in data-driven hiring?
No, AI enhances recruitment by automating tasks, but human judgment is still essential for final hiring decisions.
How does data-driven recruitment help improve diversity hiring?
It removes unconscious bias by assessing candidates based on skills and qualifications rather than demographic factors.
How can a company start using data-driven recruitment?
Start by tracking key recruitment metrics, implementing an ATS, using AI-based screening tools, and continuously optimizing hiring strategies.